TECH
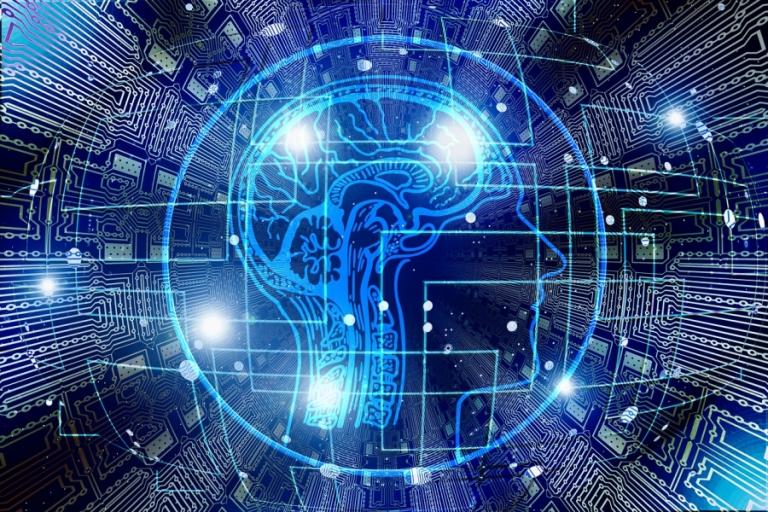
We still do not know how artificial intelligence works
How does artificial intelligence work?
Computer scientists know in general terms - very general - how neural networks develop. After all, it is they who write the training programs that direct the so-called "artificial neurons" of computers to connect to other neurons - there have been great advances in computing with neuromorphic hardware, but in actual artificial intelligence, all are mathematical functions.Each neuron analyzes information, and each neuron is based on information from previous nodes. Over time, the connections evolve. They range from random to revealing, and the network "learns" to do things like detect signs of cancer long before it is visible to a human radiologist, identify faces in the crowd, drive cars, and so on.Those are the good news.The disconcerting news is that as artificial intelligence plays an increasingly important role in human life, its learning processes are becoming increasingly obscure. Just when we really need to trust them, they have become inscrutable, they have become what computer scientists themselves call a "black box," but a black box that does not reveal their data actually conceals them from any understanding.This is a big problem."The more we rely on artificial intelligence systems to make decisions, such as driving cars autonomously, filtering news or diagnosing diseases, more importantly that artificial intelligence systems can be held accountable," argues Professor Stan Sclaroff of Boston
University , In the USA.
Creator who does not understand the creatureWhoever set out to try to get rid of this vexation that is the creator does not understand how his creature works, it was the teacher Kate Saenko, who had an idea: She asked for humans to look at dozens of photos that describe the steps that the computer can take taken on their way to a decision, and then tried to identify the most likely path the program took to reach its conclusion.Humans gave answers that made sense, but a problem arose: they made sense to humans, and humans, Saenko points out, have prejudices. In fact, humans do not even understand how they make decisions themselves. How then could they discover how a neural network, with millions of neurons and billions of connections, makes decisions?Saenko then set out for a second experiment, using computers instead of people, to help determine exactly which "cognitive roadmap" machines use to learn."This time we did not have humans on the circuit. Instead, we put another computer program to evaluate the explanations of the first program."The first program, the neural network, provides an explanation of why it made the decision by highlighting parts of the image it used as evidence. The second program, the evaluator, uses that to obscure the important parts and feeds the image obscured back in the first program."If the first program can no longer make the same decision, then the obscured parts were really important, and the explanation is good. However, if you still make the same decision, even with the obscure regions, then the explanation is deemed insufficient, "said the researcher.
Machine cognition
The team has not yet reached the cognitive roadmap of artificial intelligence. In fact, the problem is so complex that they paused to discuss which method is best to explain the decision-making process of a neural network - the human or the software?Saenko is still reluctant to choose a winner: "I would say that we do not know what is best because we need both types of evaluation, the computer has no human prejudices, so it's a better appraiser in that sense, but we continue to do the evaluation with humans in the circuit because, in the end, we know how humans interact with the machine. "So are we really doomed to rely on computer programs that we do not understand how they work?Saenko prefers to focus on more practical aspects, highlighting other questions: "Does this kind of assessment increase human confidence in neural networks? If you had a standalone car and could explain why it is driving in a way, it really would make a difference. you?""I would say 'yes.' But I would also say that we need a lot more research," he concluded.
Bibliography:Explainable Neural Computation via Stack Neural Module Networks
Ronghang Hu, Jacob Andreas, Trevor Darrell, Kate Saenko
https://arxiv.org/abs/1807.08556
No comments:
Post a Comment